The world’s population is rapidly increasing, and estimates show that under current water usage practices, there will be a 40% shortfall between forecasted demand and available supply of fresh water by 2030. Furthermore, chronic water shortages and weather extremes fueled by climate change (e.g., more frequent and harsher heat waves, droughts, etc.) are expected to pose some of the greatest threats to global prosperity and stability.
In the Middle East and North Africa, water is a scarce resource with uneven distribution among different geographic sub-regions, resulting in geo-environmental problems at the national level and conflicts between countries that share access to key rivers and water resources straddling state borders. Today, the MENA region is placing unprecedented stress on water supplies, leading to an abrupt increase in water demand, threatening local food security, and harming regional ecosystems. Most of the region is made up of arid and semiarid areas where precipitation is lower than 200 millimeters per year and evapotranspiration exceeds 55%. As a consequence, the available water resources cannot cope with human demand for all major sectors.
The stress on the region’s already limited water resources is further exacerbated by an increasing reliance on groundwater, especially in deep inland locations where access to seawater desalination is not economically feasible. Even in coastal areas, the frequent deterioration of seawater quality due to harmful algal blooms or oil spills hinders the operation of desalination plants. In such contexts, monitoring precipitation — the sole renewable water source — is essential yet also challenging due to the sporadic and unevenly distributed nature of rainfall in space and time across the MENA region.
What are the sources of precipitation data?
Estimates of precipitation amounts in all forms (e.g., rain, snow, and hail) fall into three broad categories, namely: 1) ground-based observations, 2) satellite retrievals, and 3) climate/weather model runs. While output products from global climate models (GCMs) and Earth system models (ESMs) provide worldwide coverage of water cycle components, their relatively coarse spatial and temporal scales limit their value for local-scale monitoring. Hence, remotely sensed precipitation retrievals from satellite platforms offer an attractive alternative to model products due to their higher spatiotemporal resolutions and continuous coverage.
When it comes to reliable, long-term, and global precipitation products, only a limited number are currently available:
-
Tropical Rainfall Measurement Mission (TRMM) products,
-
Global Precipitation Measurement (GPM) mission products,
-
Global Precipitation Climate Center (GPCC) products, and the
-
Climate Hazards Group InfraRed Precipitation with Station data (CHIRPS) product.
The global long-term spatial patterns of annual precipitation were found to be in agreement between the above products (and others), but they often reveal marked differences regionally.
What are the region-specific challenges for precipitation monitoring over MENA?
The disagreement among satellite-based precipitation products has not been thoroughly studied for the MENA region. The lack of study is particularly critical for the Arabian Peninsula, where relative error margins can reach up to 300% between different satellite-based precipitation products.
This discrepancy is primarily attributable to the scarcity of dense and reliable ground observations from rain gauge networks and the absence of large urban developments in arid and hyper-arid regions. The predominant desert land cover across the Arabian Peninsula is also especially problematic — the high land surface reflectivity from deserts introduces errors in the microwave-based precipitation estimation algorithms (similar reflectivity-induced errors are also evident over the polar ice caps).
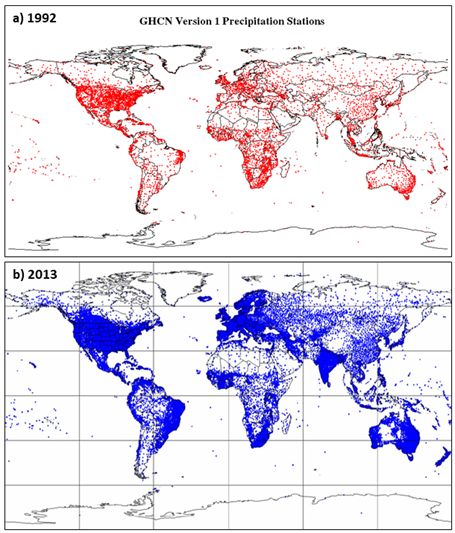
The sparse distribution of rain gauges and inhomogeneity of observations hamper the calibration of such products over the MENA region. Although most parts of the world saw an expansion in the number of rain gauge networks over the past decade, the MENA region continues to suffer from the lowest density of these — the figure on the right shows the global distribution of rain gauges included in the Global Historical Climatology Network in 1992 and 2013. While increasing the availability of data from rain gauges is a crucial step for improving continuous precipitation monitoring, this is only the tip of the iceberg.
Most Earth observation satellite missions are launched and managed by long-standing national space agencies, namely NASA, ESA, and JAXA. This explains why data products from orbital missions produce reliable precipitation estimates over the United States, Europe, and (most of) Asia but continue to exhibit large inaccuracies over other regions, like MENA. Regional participation of MENA countries in Earth observation satellite missions will most certainly draw more attention to improve satellite acquisitions in this understudied area of the world.
In 2006, the Mohammed Bin Rashed Space Center (MBRSC) began the development of the United Arab Emirates’ first satellite, and a team of Emirati engineers traveled to South Korea as part of a knowledge transfer program. Over the next decade, working with South Korean experts, the MBRSC’s satellite engineers designed and manufactured the country’s first nanosatellite, Nayif-1, and its first two observation satellites, DubaiSat-1 and DubaiSat-2, which were launched in 2009 and 2013, respectively. In October 2018, the launch of the first 100% UAE-designed and manufactured Earth observation satellite, the highly sophisticated KhalifaSat, was celebrated as a historical milestone. KhalifaSat cemented the country’s position among the world’s leading space technology manufacturers and its role in actively contributing valuable satellite imaging data to the international scientific community. More Arab countries, including Saudi Arabia and Qatar, have been vocal about their ambitions to join the space-borne Earth observation community. This may lay the foundation for a Gulf Cooperation Council (GCC)-wide space program that includes satellite-based Earth observation as one of its strategic pillars.
AI-driven reverse hydrology solutions
Satellite-based precipitation bias correction and multisource estimation approaches have been explored and applied to different regions. It is important to distinguish between 1) the conventional approach of exclusively relying on rain gauge (ground) observations and 2) the more recent approach of incorporating additional explanatory variables to correct precipitation estimates.
Remote sensing technology can provide valuable information about water resources, such as surface water, groundwater, and soil moisture — all proxies for precipitation estimates. For example, the water content in the topsoil layer directly corresponds to rainfall and is widely used as evidence of an observed rainfall event. In fact, most satellite-based soil moisture retrieval algorithms are crosschecked using precipitation flags (rain/no rain) from available precipitation data sources. This soil moisture-precipitation dependency is particularly relevant for arid regions and desert environments, where background soil moisture before a rain event is low due to negligible surface flow and water bodies such as rivers and lakes. Therefore, soil moisture variations are controlled by rainfall occurrences and provide a sustained surface signature beyond the satellite overpass time (e.g., the 30-minute time interval for the GPM product).
Desert regions also experience unique physical processes that are specific to arid regions. The predominant sandy and dry soil cover can quickly drain any surface water from rainfall to the deep aquifers. Alternatively, rainfall also compacts these sandy soils, making them less conducive to surface water drainage. Accounting for these physical processes is critical when dealing with surface soil moisture retrievals from Earth observation satellites.
To account for these processes and improve the use of remote sensing for water resource management in the Middle East, it is necessary to include more explanatory variables in the processing of satellite-based precipitation products, such as soil type, evapotranspiration, and vegetation cover. Additionally, a combination of remote sensing, model reanalysis, and ground-based measurements, known as “reverse hydrology,” can improve the accuracy of water resource data. Artificial intelligence (AI) and machine learning tools are essential for managing these large datasets.
The first attempt to assess the consistency of different precipitation products, specifically over the Arabian Peninsula, is outlined in a recent study, which employed advanced statistical techniques to infer water storage variations from inputs of soil moisture, terrain elevation, and four different satellite-based precipitation datasets. The GPM IMERG product recorded the highest consistency with soil and water storage variables, rendering it the most promising global satellite-based precipitation product for the MENA region.
Spotlight on promising research progress
Blending explanatory variables to enhance precipitation estimates has been increasingly applied using Artificial Neural Networks (ANNs), a subset of machine learning techniques that perform adaptive, efficient, and holistic mappings of nonlinearities between large datasets.
In 2020, the first attempt was conducted for ANN-driven precipitation bias correction over the UAE by correcting the GPM IMERG’s latest daily product overland using ancillary data and explanatory variables. The below figure illustrates the proposed configuration of the ANN model, which uses soil moisture and terrain elevation datasets and local precipitation estimates from a radar network. The model derives a single output from these variables, which should (ideally) match the observed rainfall amounts recorded by the rain gauges. Model testing showed error measure reductions of 56% (and 25%) for GPM estimates and 34% (and 53%) for radar estimates during summer (and winter) periods. Details on the proposed model architecture, calibration, and training/testing can be found in the published study. While the ANN merging technique was developed locally for the UAE’s precipitation estimation, the proposed methodology applies to other arid regions requiring improved precipitation monitoring.
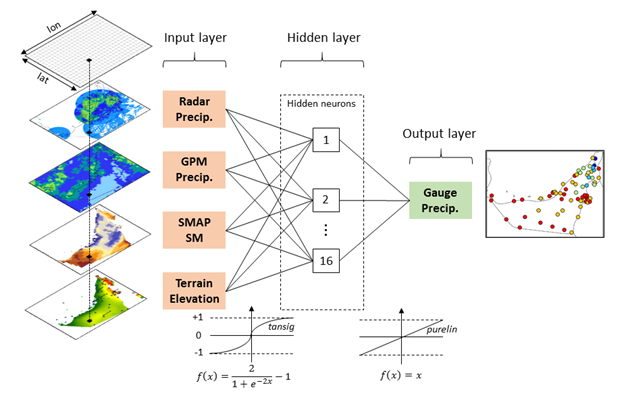
The UAE Research Program for Rain Enhancement Science (UAEREP) is an international merit review research initiative established in 2015 to stimulate and promote scientific advancement and the development of new technology in the field. Since its inception, the UAEREP has provided managed grant assistance to projects targeting innovative research in atmospheric sciences, focusing on innovative water security solutions.
One of the program’s latest funded projects in 2022 is entitled “A Hybrid Machine Learning Framework for Enhanced Precipitation Nowcasting.” This project assembled a multidisciplinary and diverse research team led by the Scripps Institution of Oceanography at the University of California San Diego with collaborators from Khalifa University (UAE) and Colorado State University.
This project aims to create an AI research and operations testbed in the UAE. Completing this project entails building a novel AI framework to blend satellite observations, ground-based weather radar data, rain gauges, and numerical weather prediction estimates to extract features and generate products to generate more accurate quantitative precipitation estimates in this region. A proposed advanced deep learning algorithm will be fed thousands of examples from historical data on how to effectively extract and extrapolate inputs and the required data features to produce more accurate precipitation estimates. These features and inputs, along with extrapolated satellite and radar data, numerical weather prediction data, and rain gauges, are utilized as inputs to an AI-based model to generate precipitation predictions six hours in the future.
The main deliverable at the end of the project will consist of a prototype of AI-based predictive capabilities. The prototype will be in use at the National Center for Meteorology (NCM) in the UAE through a research and operations partnership. This will offer a tangible outcome from international research collaboration to advance our understanding of weather and climate.
Dr. Youssef Wehbe is a Non-Resident Scholar with MEI’s Climate and Water Program and a program officer with the NCM.
Photo credit: Julian Herzog/NASA via Wikimedia Commons
The Middle East Institute (MEI) is an independent, non-partisan, non-for-profit, educational organization. It does not engage in advocacy and its scholars’ opinions are their own. MEI welcomes financial donations, but retains sole editorial control over its work and its publications reflect only the authors’ views. For a listing of MEI donors, please click here.